AI Bias: The Comprehensive Overview and Mitigation Strategies
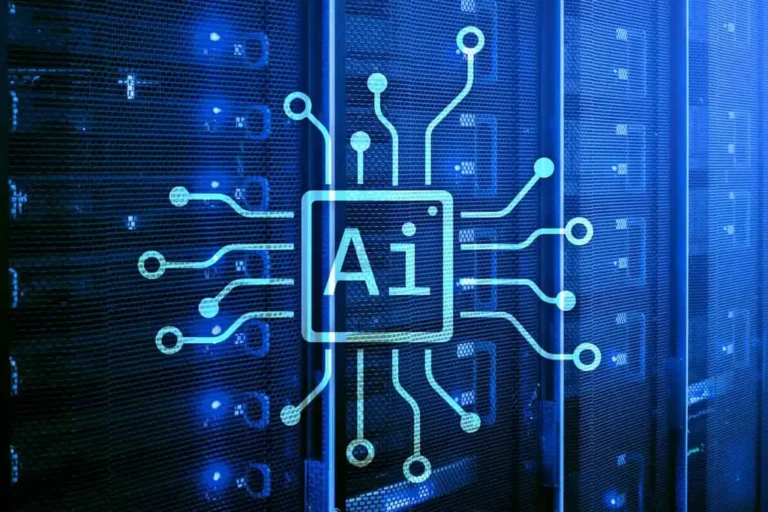
The development of Artificial Intelligence (AI) technology led to the proliferation of solutions based on Large Language Models (LLMs). Companies deploy such tools to automate processes, minimize downtime, and serve customers 24/7. By integrating recommendation systems into their platforms, businesses provide personalized client experience. Training AI models requires using high-quality datasets. Even minor issues can cause serious consequences, resulting in substantial fees and compliance issues. In this guide, we will analyze the impact of AI bias on business operations and consider how to improve the quality of source data used by companies.
What is AI Bias?
The term refers to flaws in the source data resulting in the poor-quality output. If training data contains biased information, it distorts the results generated by AI algorithms. This phenomenon is similar to AI hallucination cases. An LLM generates misleading replies due to the insufficient amount of data or its low quality.
Bias makes AI models less accurate and reliable, thus hindering technology adoption. If such issues remain unsolved, they disrupt a company’s operations and make it more challenging to win clients’ trust. The artificial intelligence bias may have a disastrous effect on a firm’s relationships with marginalized communities.
LLMs trained on biased data fail to produce information that could help underprivileged groups. When integrated with existing systems, such AI models fail to ensure societal equity while screening candidates’ CVs or calculating credit scores. Even facial recognition software is biased, as it has lower accuracy in analyzing the visuals of dark-skinned people. As the usage of AI becomes more widespread, learning how to recognize the signs of bias will be essential to maintain an edge over competitors.
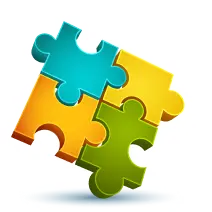
We are confident that we have what it takes to help you get your platform from the idea throughout design and development phases, all the way to successful deployment in a production environment!
AI Bias Examples
The manifestation of algorithmic bias results in a host of consequences. Low-quality AI systems fail to treat clients equally. As a result, a company’s image suffers. Below, we have outlined the industries where prejudice can cause a lot of harm:
- Healthcare: A limited amount of data about women’s health issues or problems faced by minorities makes an AI algorithm unreliable and hinders decision making. The usage of computer-aided diagnosis solutions may be less effective due to machine learning bias. ML technology could aggravate existing health inequalities and worsen the effect of prejudice. LLMs trained on incomplete data deliver subpar performance when providing solutions for underrepresented communities.
- Human resources: While AI-driven recruiting tools make it easier for agents to scan through resumes and select suitable candidates, they prevent underprivileged people from advancing to the interview stage. Besides, they may demonstrate an age bias or hire more men than women. When Amazon developed its complex hiring system with an automated credit scoring mechanism, it exemplified the problem of bias in AI. This system failed to screen candidates using a gender-neutral approach. Even though the company took the necessary steps to change its software, detecting other inherent stereotypes proved to be more challenging.
- Top management: Generative AI bias becomes noticeable if LLM-based systems prefer to portray typical CEOs as males. For instance, the advertising system used by Google may display information about high-paying job offerings mostly to males.
Improving the quality of source data allows companies to win clients’ loyalty and build a positive brand image.
Types of AI Bias
Distorted information affects a company’s operations. Testing data to check its accuracy is necessary to avoid performance issues and resource wastage. Learning how to recognize different types of bias allows firms to deal with them efficiently:
- Algorithm bias: If a person does not ask a specific question, an AI model may provide an irrelevant answer.
- Cognitive bias: The creators of datasets and AI models often fail to detect specific biases, as they do not consider themselves prejudiced.
- Confirmation bias: When AI tools are built based on the current data trends, the information quality degrades.
In some cases, essential info gets omitted because developers fail to recognize its importance. The out group homogeneity bias is present in the situations when people have limited knowledge about the representatives of other populations. Some ventures also have to deal with measurement issues, when they fail to include a specific dataset into research. The failure to maintain consistency during data labeling also leads to inaccurate results.
How to Avoid Bias
Companies that want to maintain a positive image and ensure that their products and services are accessible to all groups strive to improve the quality of data used by their custom LLMs. Here are the steps they take to avoid biases:
- Choose the right LLM. Utilizing human-in-the-loop technology allows ventures to fine-tune AI models and train them using relevant data.
- Create a team. It should include people from different backgrounds to ensure that they can recognize the signs of bias.
- Focus on data processing. Eliminating low-quality data allows ventures to create reliable datasets.
- Perform regular monitoring. This approach enables ventures to detect bias in time. Some enterprises hire third-party service providers to monitor their operations.
In addition, companies should maintain their equipment in order and repair mechanical sensors in time to get accurate data and build powerful LLMs.
Final Thoughts
Further AI development is expected to make LLMs more reliable. Eliminating AI bias allows companies to provide top-quality service catering to the needs of diverse populations. Even though it may be impossible to achieve full impartiality, ventures should focus on maintaining transparency and accountability. The Global Cloud Team includes experts specializing in training advanced LLMs based on high-accuracy data. Our professionals develop complex systems that deliver impartial outputs. Trained to recognize various AI biases, we create algorithms that can automatically correct issues. Get in touch with our team today and discover how to deploy the best practices to collect accurate data for AI models.
Top Articles
AI Platform as a Service: Main Components and Applications
I am here to help you!
Explore the possibility to hire a dedicated R&D team that helps your company to scale product development.

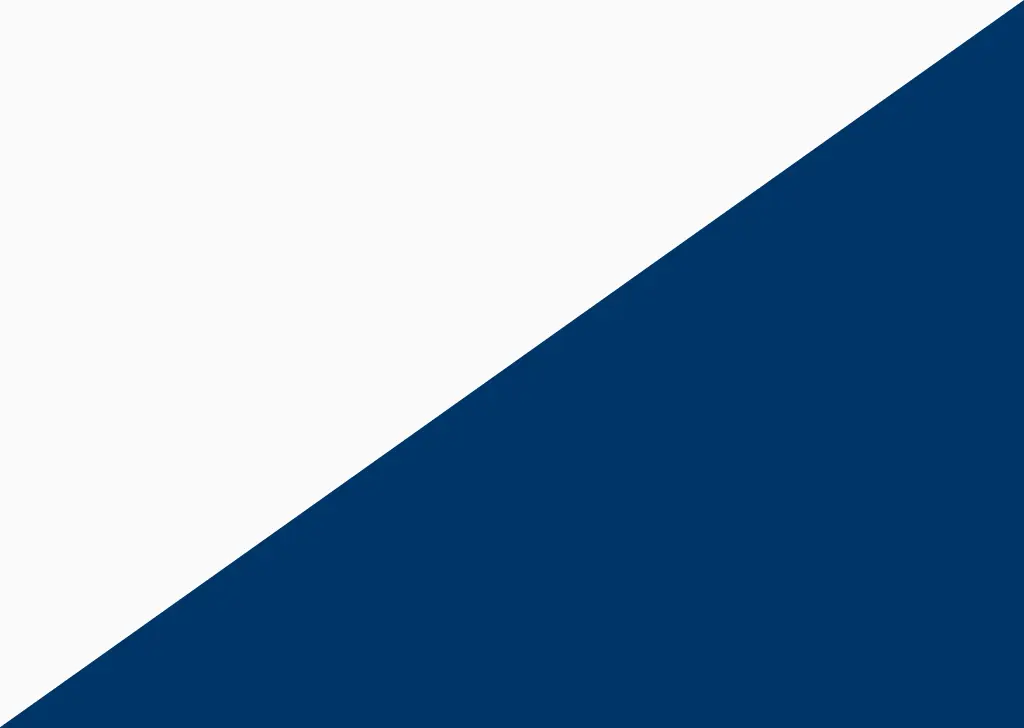