Explainable AI: Advantages and Applications of XAI Practices
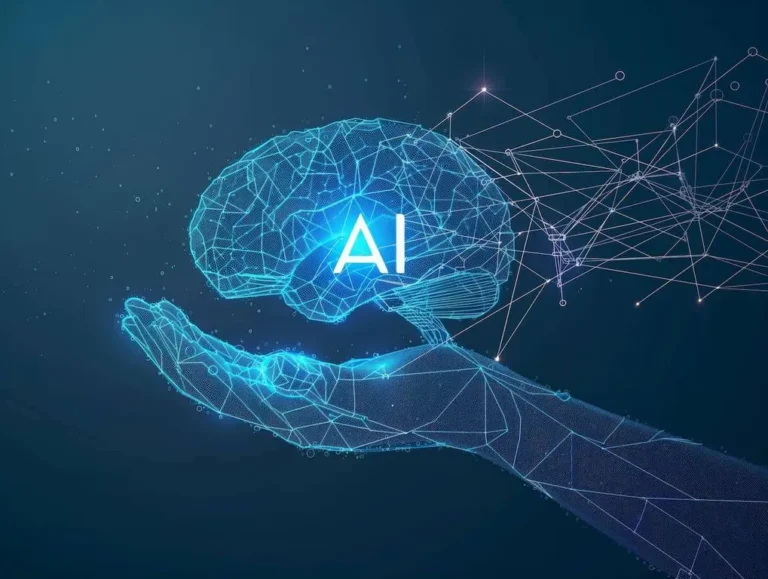
The development of artificial intelligence (AI) technology enables businesses to improve their operational efficiency. However, sometimes the decisions made by automated systems are impossible to comprehend, which hinders decision making. Lack of trust in AI is one of the main barriers preventing its widespread adoption. In this article, we will examine the concept of explainable AI (XAI) and demonstrate its pivotal role in the sectors that prioritize transparency and accountability. Mastering methods that restore confidence in AI is crucial for firms that want to integrate such tools with legacy systems and get an edge over competitors.
What is Explainable AI?
The term XAI refers to techniques that make outputs generated with the help of machine learning (ML) tools and algorithms easier to understand. It encompasses various approaches to describing large language models (LLMs), the impact of AI, and the inherent biases. The market is predicted to reach $21.06 billion by 2030.
Focusing on accuracy and transparency, XAI becomes the only way to analyze the steps followed by an algorithm. The black box concept implies that it is impossible to fully grasp how a bot comes to a specific conclusion. However, XAI signifies a pivotal step in the way of understanding algorithms better.
XAI allows developers to confirm that the tools they build function as they want them to, besides, it facilitates meeting regulatory requirements, and simplifies the process of fine-tuning LLMs to achieve the set objectives.
While the black box model describes the relationship between the input data and output, XAI focuses on what happens in between, making it invaluable in the healthcare and finance industries. Without disregarding the seeming ungraspability of algorithms, XAI techniques allow users to interpret their internal processes.
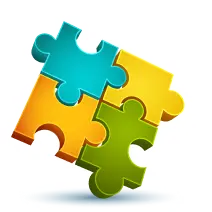
We are confident that we have what it takes to help you get your platform from the idea throughout design and development phases, all the way to successful deployment in a production environment!
The Main Principles of Explainable AI
XAI enables companies to gain insights into AL technology and understand how algorithms make specific decisions. It facilitates making the necessary adjustments to refine an LLM’s performance and empowers firms to enhance user experience (UX) and ignite confidence in AI.
Keeping track of ML processes lets enterprises streamline the functioning of LLMs and boost the accuracy of outputs. XAI techniques are based on three principles:
- Prediction accuracy: Launching simulations allows employees to see whether XAI outputs are similar to the results they receive using training data. Using the Local Interpretable Model Agnostic Explanations (LIME), it becomes possible to offer interpretations of a prediction.
- Traceability: This method facilitates achieving XAI by limiting the ways an algorithm can make specific decisions. The DeepLIFT technique involves comparing activated neurons to references and demonstrating dependencies between them. By leveraging this method, businesses get insights into ML rules.
- Understanding decisions: Due to the widespread mistrust in algorithm-driven systems, firms deploy explainable artificial intelligence techniques to demonstrate how to use such solutions. Employees should see how AI comes to conclusions to learn how to make the most out of LLMs.
These principles highlight the significance of XAI and show that this concept opens a pathway to increased adoption of new technologies.
Why Utilize XAI?
Besides inspiring confidence in algorithms, XAI fosters transparency and contributes to the increasing usage of AI. There are multiple reasons to embrace such procedures:
- Promote regulatory compliance: Authorities prefer to see whether a specific product was built per existing standards and guidelines. In Europe, GDPR emphasizes the importance of disclosing information about the inner workings of automated processes. Due to XAI, companies remain compliant and follow local policies to shed light on the logic behind the decisions made by automated systems.
- Bolster accountability: When ML models generate outputs that are difficult to explain, it becomes crucial to analyze what factors they take into account. XAI expedites discovering the causes of faulty decisions and lets firms take prompt steps to fix mistakes.
- Build trust: High-risk industries like healthcare should demonstrate the reliability of ML tools to stakeholders. XAI helps employees show how such services can enhance patient journeys.
- Optimize performance: Improving explainability in AI allows experts to configure models to bolster their efficiency and ensure that they produce accurate outputs.
- Tighten control: Unveiling potential vulnerabilities enables organizations to eliminate potential weaknesses and enhance the overall efficiency of AI systems.
Due to these advantages, XAI approaches become widely used by companies that want to streamline governance, improve collaboration, and ensure that their LLMs make result-yielding decisions.
Use Cases of Explainable AI
Due to the extensive applicability of artificial intelligence technologies, XAI can be used across many industries. Let’s consider the main sectors where such methods help stakeholders gain confidence in AI.
Healthcare
This industry is predicted to benefit from the implementation of automated systems. However, as it has strict regulations, responsible AI usage is necessary to make the most out of the new technology. Health professionals utilize XAI techniques to perform the following tasks:
- Explain anomalies: Using XAI, professionals can see why automated tools flag specific X-rays. Thus, doctors make decisions based on facts and achieve better patient outcomes.
- Categorize threats: XAI enables specialists to learn why an LLM indicates that a specific patient has an increased risk of developing a disease. It facilitates taking the right steps to improve a patient’s health.
- Make drug prescriptions: Using XAI, experts explain why AI tools suggest taking a specific drug. They enable doctors to provide timely treatment recommendations.
- Clinical trial design: XAI methods enable specialists to check what criteria complex systems use when selecting candidates for clinical trials.
The effective use of explainable AI tools allows organizations to boost the quality of the provided services.
Finance
Bank employees need to make quick decisions after analyzing a transaction history. Processing large volumes of data requires using algorithm-driven solutions. Financial organizations deploy XAI to do the following:
- Shed light on the factors impacting loan denial or approval;
- Unveil inherent biases;
- Assess compliance;
- Detect signs of fraud;
- Analyze creditworthiness and potential risks;
- Check investment recommendations generated by virtual assistants.
Similarly, insurance companies deploy XAI to clarify why specific claims were approved or denied. They check whether LLMs were built according to ethical guidelines.
Automotive companies
The increasing popularity of autonomous vehicles (AVs) and the usage of AI in the industry highlight the importance of XAI. Using such tools, experts explain why AVs follow a specific course of action when facing an obstacle.
Machine learning operations (MLOps) in the manufacturing sector facilitate automating data testing and validation, thus contributing to the reliability of ML models. They enable organizations to embrace systematic methods of achieving XAI. Maintaining transparency helps businesses to foster trust and explain the functioning of advanced driver-assistance systems.
With XAI, firms achieve such goals:
- Identify hazards;
- Discover the system’s limitations;
- Improve maintenance and avoid costly repairs.
Gaining a deeper understanding of the functioning of AVs is the first step toward responsible use.
Other industries
Law firms adopt XAI procedures to check why digital assistants focus on specific documents during e-discovery. It makes legal proceedings transparent and ensures that important data won’t get overlooked.
Travel agencies explain the reasons behind recommendations provided by bots and clarify how destinations are selected based on a client’s history. XAI allows stakeholders to see how algorithm-based tools choose the best deals and provide other services.
Mastering Explainable AI for Business Growth
Recognizing the significance of XAI lets businesses leverage innovative practices to improve transparency and accountability. Making such principles a basis of their governance practices permits companies to achieve sustainable growth. Firms should take such steps to implement XAI:
- Create a governance committee: This step includes recruiting members and outlining their responsibilities.
- Set standards: When training AI models, it’s crucial to understand whether employees will be able to explain their decisions in different contexts. Besides, firms should set escalation guidelines.
- Create tools to help developers assess risks: The team should deploy tools designed to monitor the performance of algorithm-based systems.
- Invest in research and development (R&D): Using explainable AI practices requires hiring experienced professionals and launching educational programs for current employees.
Successful companies understand the importance of retaining experts who have deep knowledge of technologies and know how to adhere to regulatory requirements to avoid legal issues. Besides, it is crucial to pay for more advanced tools to process large datasets with better accuracy and provide relevant explanations.
Implementing XAI practices may be expensive and time-consuming without any prior experience. This is why companies often outsource this task to trusted service providers. While initial investments in ready-made solutions may seem high, they are more feasible in the long run and have more applications than open-source tools.
Conclusion
Due to deep learning technology, LLMs are capable of improving their performance. However, without human supervision, they may magnify the existing biases and offer unjust solutions. Boosting stakeholders’ confidence in AI assistants’ decisions requires using XAI approaches to emphasize the reliability of AI-generated outcomes. Even though simplifying bots may foster trust, process optimization may affect the accuracy of such solutions.
Explainable AI methods unveil the main factors impacting the system’s decisions. Firms utilize dedicated tools to avoid compromising quality and automate processes without breaking ethical norms. The Global Cloud Team specializes in creating advanced software solutions for companies from different industries. Contact our professionals today and learn how to use XAI to build well-performing LLMs with comprehensible outputs.
Top Articles
SOA vs Microservices: An Overview of the Main Differences
I am here to help you!
Explore the possibility to hire a dedicated R&D team that helps your company to scale product development.
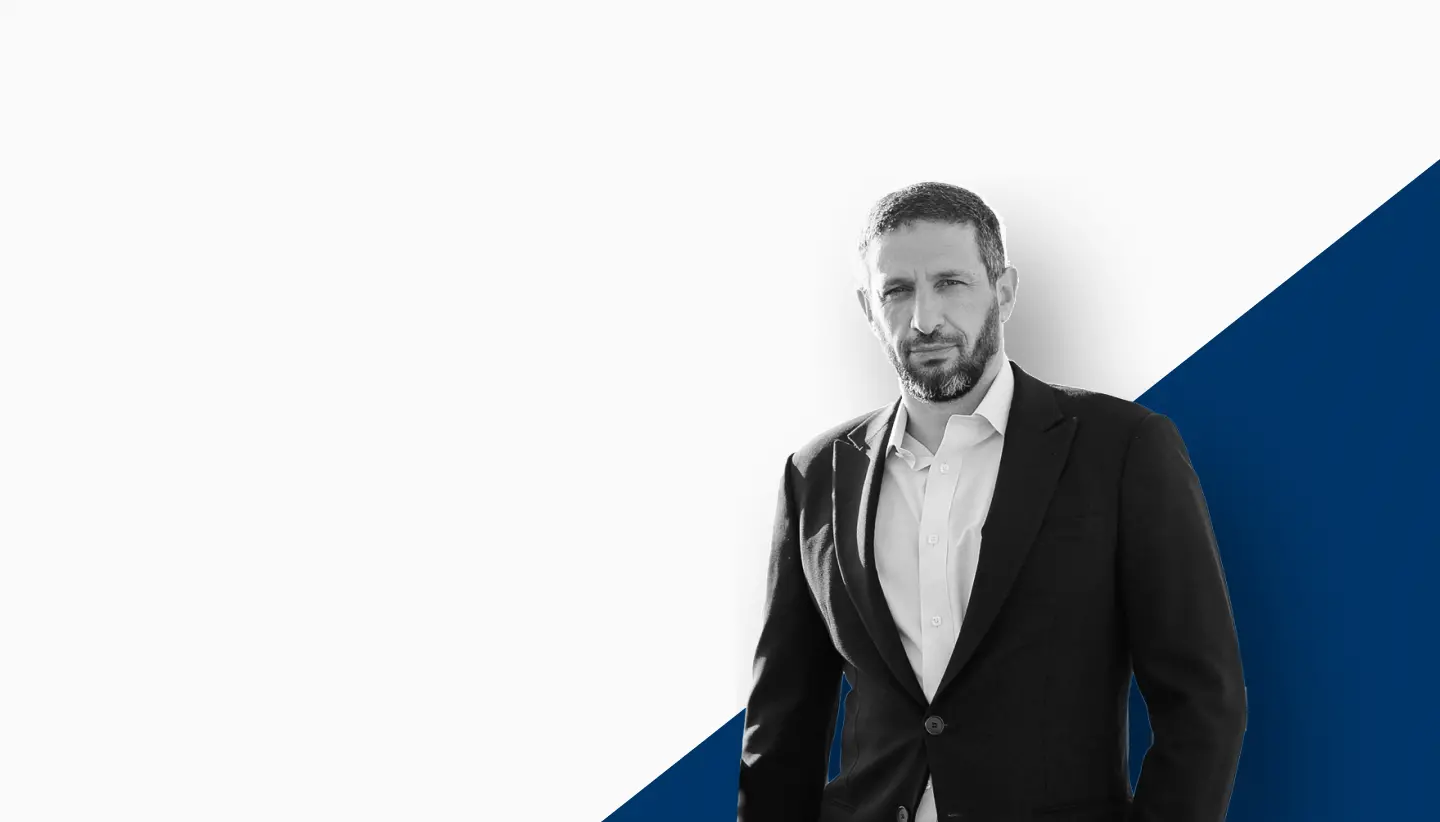
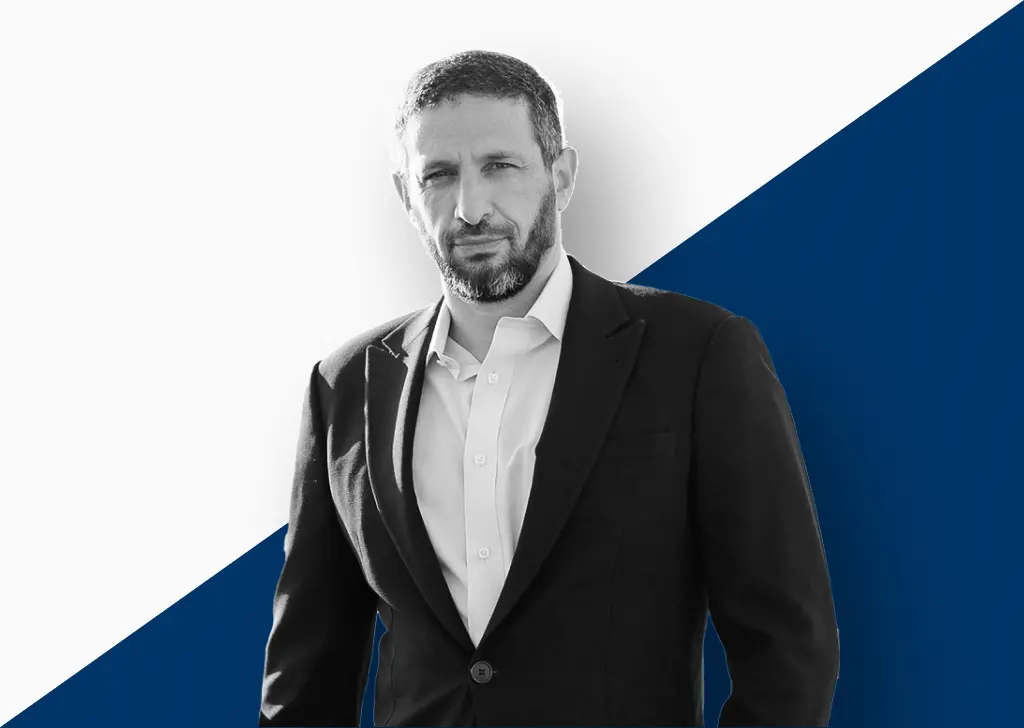