A State of the Art Human Detection AI Algorithm
The Task
We present a state of the art model that can detect humans much better than any other existing model, by using advanced research and new techniques our model detects humans even in very dense environments, and counts them correctly.
Figure 1. Qualitative results produced by our algorithm with the ground truths, ie, real number of people.
Crowd counting plays a vital role in surveillance, public safety, and managing large gatherings, with numerous strategies proposed for estimating crowd sizes. These include direct counting, density estimation, object detection, and point localization, with recent state-of-the-art methods often utilizing density estimation and point localization.
One alternative approach involves employing generative models to predict crowd density maps, learning the distribution of density values within these maps. Although Generative Adversarial Network (GAN)-based models have been utilized for this purpose, they tend to use large kernel sizes and do not fully take advantage of point supervision. Using a large kernel can hinder the model’s ability to maintain the diversity of density pixel values. Moreover, the potential of combining point supervision with generative models for crowd density prediction has been relatively unexplored. Current GAN-based models typically produce a single density map output, overlooking the generative models’ capability to create multiple density map outcomes, which could enhance counting accuracy.
Our research focused on using denoising diffusion probabilistic models (diffusion models) to generate accurate crowd density maps from images. By incorporating a narrow Gaussian kernel in the ground truth density maps used for training, we reduced the overlap between adjacent densities. This approach not only preserves the distribution of density pixel values but also facilitates the diffusion model’s learning process, resulting in more precise density predictions. Our method successfully replicated the narrow kernel in densely populated areas, outperforming other models that did not.
To address potential density loss in density-based crowd counting methods, we developed a technique that counts the blobs in the predicted density map by identifying contours. This method bypasses the need to aggregate density pixel values, thus reducing background noise interference. We further introduced a crowd map fusion strategy that merges multiple dot maps created from contour detections, leveraging the stochastic nature of generative models to boost counting accuracy. Additionally, an auxiliary regression branch is used during training to estimate crowd sizes from the denoising network’s encoder-decoder features, enhancing feature learning.
Embrace innovation with Global Cloud Team’ bussiness competence and services
Table 1. Comparison with the SOTA techniques on JHU-CROWD++, ShanghaiTech A, ShanghaiTech B, UCF CC 50, and UCF-QNRF datasets. The best results are highlighted in bold.
Method | JHU-CROWD++ | ShanghaiTech A | ShanghaiTech B | UCF CC 50 | UCF-QNRF | |||||
MAE | MSE | MAE | MSE | MAE | MSE | MAE | MSE | MAE | MSE | |
ADSCNET | – | – | 55.40 | 97.70 | 6.40 | 11.30 | 198.40 | 267.30 | 71.30 | 132.50 |
SUA | 80.70 | 290.80 | 68.50 | 121.90 | 14.10 | 20.60 | – | – | 130.30 | 226.30 |
SASNet | – | – | 53.59 | 88.38 | 6.35 | 9.90 | 161.40 | 234.46 | 85.20 | 147.30 |
ChfL | 57.00 | 235.70 | 57.50 | 94.30 | 6.90 | 11.00 | – | – | 80.30 | 137.60 |
MAN | 53.40 | 209.90 | 56.80 | 90.30 | – | – | – | – | 77.30 | 131.50 |
GauNet | 58.20 | 245.10 | 54.80 | 89.10 | 6.20 | 9.90 | 186.30 | 256.50 | 81.60 | 153.70 |
CLTR | 59.50 | 240.60 | 56.90 | 95.20 | 6.50 | 10.60 | – | – | 85.80 | 141.30 |
Ours | 49.17 | 202.91 | 51.62 | 85.68 | 6.03 | 9.40 | 155.38 | 216.59 | 62.15 | 124.36 |
Team
We have extensive experience in the development of highly scalable robust distributed platforms. As an example, the largest project was developed by multiple collaborating Outstaff Teams within GCT employing over 70 engineers.
The developed financial services platform supports up to 5 thousand updates per second and serves millions of end-users.
We believe that it takes great people to deliver a great product.
I am here to help you!
Explore the possibility to hire a dedicated R&D team that helps your company to scale product development.

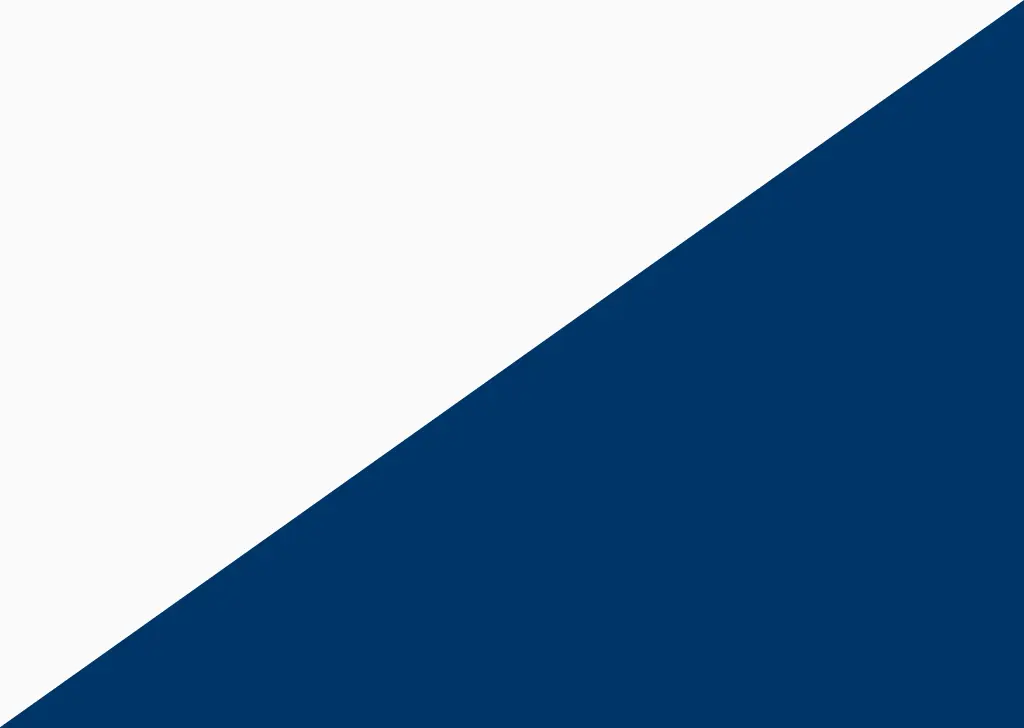
Our scalable workforce is specializing in the following areas of software development

Revolutionize manufacturing processes and increase productivity with our innovative software solutions
When it comes to developing software for the financial sector, cooperate with GlobalCloudTeam
We have the skills, experience, and resources to develop even the most complex healthcare solution
Strengthen your market position with GlobalCloudTeam eCommerce solutions
Drive innovation in the automotive industry with cutting-edge software development services from GlobalCloudTeam
Explore our solutions
