The Main Aspects of Artificial General Intelligence (AGI)
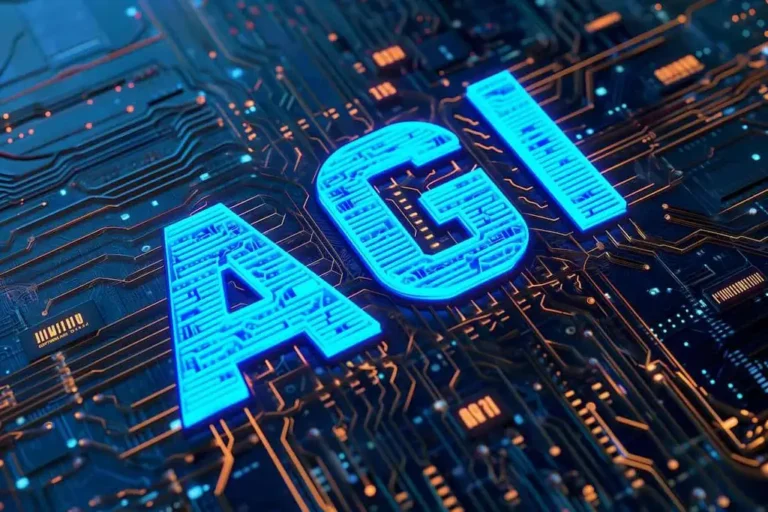
Not long ago, the world faced the next frontier: artificial general intelligence (AGI). It has significant potential and surpasses standard artificial intelligence (AI) to achieve something big. The main goal of AGI is to build computers that may not just imitate live agents’ actions but actually realize them, offer unique concepts, and utilize the knowledge gained. It is not just about creating unique devices but about transforming what we call intelligence. Below, we will discuss the primary elements of AGI and its prospects.
The Main Aspects of AGI
Artificial general intelligence (AGI) is a form of theoretical AI-based solution that aims to produce AI with human-level cognitive capabilities, including learning independently. However, not all AI professionals are confident such a system is possible. Furthermore, ongoing discussions focus on determining the appropriate factors to accurately measure “intelligence.”
Other terms are also used to define AGI, including strong AI or full AI. Such a system format fundamentally differs from weak AI and narrow AI. The latter can only perform particular operations within the approved parameters. AGI may solve many troubles independently in various areas of human life.
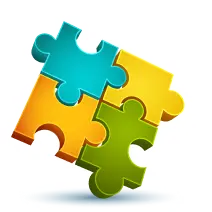
We are confident that we have what it takes to help you get your platform from the idea throughout design and development phases, all the way to successful deployment in a production environment!
What Is AGI Capable of?
Real AGI must cope with human-level jobs that no modern PC can handle. AGI solutions must have some capabilities and characteristics:
- abstract reasoning
- experiential learning
- intuitive understanding
- discernment of cause and effect relationships
- ability to transfer acquired knowledge
Let’s look at the primary features of AGI:
- Creativity. An AGI solution would, in theory, be able to interpret, understand, and enhance code written by engineers.
- Sensory perception. AGI could recognize colors, which is a subjective assessment. It will also be able to identify the three-dimensionality of pictures.
- Optimal motor skills. One example is pulling keys out of your pocket, which requires creativity.
- Navigation. We are accustomed to using the Global Positioning System to understand our location. Once implemented, AGI systems may create motion projections in the environment better than other known systems.
AI developers expect AGI solutions to gain higher-level abilities, such as engaging in metacognition and utilizing a metacognitive database.
Distinctions between AGI and AI
The primary distinction between AGI vs AI is their potential and level of generalization. Let’s discuss their main characteristics.
Narrow AI (aka “weak AI”) is the smart solution we interact with today. It allows limited tasks, including image recognition, language translation, and textual content generation. Although AI-backed systems excel at these activities, they cannot extend their knowledge to other fields or solve problems for which they were not trained.
Artificial general intelligence or strong AI is a hypothetical category of AI with human-like intelligence in various areas. AGI may reason, learn, plan, interpret words correctly, and solve issues as a human would. It can utilize its knowledge and skills in non-standard situations and adapt to dynamic conditions.
As we know, modern AI-backed instruments are specialized tools that help solve specific troubles. Simultaneously, AGI is a one-stop solution to any problem. It can learn to solve any intellectual problem people can handle.
Technologies at the Heart of AGI
AGI is still a promising area for research. Efforts to develop AGI solutions do not stop; new developments are constantly appearing. Below, we describe the main tools to activate growth.
- Deep learning (DL) is a subfield of machine learning (ML) that simulates the connections in the human brain through neural networks. Its neural systems include model layers to define patterns in the selected database. They use significant information for precise training, which requires powerful hardware, including GPUs and TPUs.
- Generative AI (genAI) is a kind of DL that allows AI-backed systems to compose unique content based on past-acquired data. GenAI solutions are trained using significant datasets. They may generate text, audio, and pictures as if a human had created them.
- Natural language processing (NLP) is an AI-backed technology that understands human language and reduces the need to cooperate with the screen. AI-ruled instruments transform human speech into computer code, which may be harnessed to run various applications.
- Computer vision (CV) is a tool that allows AI-backed systems to collect, process, and interpret spatial insights from visual databases. Self-driving automobiles utilize CV to process information from cameras in real-time and drive safely, avoiding all obstacles. With DL, computer vision instruments can recognize, classify, track, and perform other image-processing procedures.
- Robotics is a technology that creates mechanical systems to perform physical manipulation. At AGI, robotic solutions enable ML to manifest itself physically. Realizing the potential of the sensory perception and physical processes AGI solutions require is essential. So, an AGI robot can sense, touch, and peel a banana, just like people do.
The creation of AGI involves a mix of various technologies and approaches. The purpose is that such smart systems will eventually be able to reason, solve troubles, realize complex concepts, and actively cooperate with the environment.
Categories of AGI Research
The search for AGI covers different techniques, each with its algorithms and rules for realizing intelligence.
- Symbolic. This technology suggests we can make solutions smart by providing them with much information about signs, norms, and logical connections. Simply put, it is necessary to divide human thinking into separate components and train PCs to think by adding these elements. Back in the 80s, medical diagnostic systems arose based on this concept.
- Emergentist. This concept posits the emergence of intelligence involves intricate, dynamic cooperation within a system comprising simpler elements. It is closely related to neural networks and DL. A striking sampler is Google’s DeepMind organization, which created AlphaGo. It mastered the game of Go not via programmed standards but through self-teaching.
- A universal approach. This technology focuses on solving AGI problems at the computational level. They generate theoretical systems that may be integrated into practical AI-backed solutions.
- Hybrid technology combines the best elements of multiple technologies to form powerful and flexible AI solutions. Proponents of such a technique recognize that perhaps no single path leads to AGI, but together, they will achieve their purposes. A famous sampler is BM Watson, which combines NLP, data mining, and rule-based reasoning.
Such exciting concepts seek to realize the multifaceted character of humans and artificial intelligence.
The Advantages of AGI
The expected profits of AGI are enormous. They may revolutionize various aspects of human life. Although this is still just a theory, AGI could bring the following benefits:
- They are solving the world’s primary troubles. AGI’s unique cognitive characteristics allow it to process significant information, look for patterns, and formulate innovative solutions to complex issues, including climate change, pandemics, and resource shortages.
- Scientific and technical progress. AGI can accelerate research in various fields, including medicine, energy, space, etc. This could lead to breakthroughs in creating drugs, improving the energy sector, and conducting complex experiments.
- Maximum efficiency. AGI automates activities across sectors, increasing efficiency, decreasing human error, and freeing up live agents to concentrate on creative activity.
AGI may help solve problems such as low income, inequality, and environmental pollution. This is possible through the optimization of resource allocation, the formation of sustainable solutions, and the cooperation of all countries.
Primary Difficulties with AGI Development
Developers must solve several more problems before creating AI solutions that are often featured in sci-fi TV series. Let’s examine the main issues current AI-ruled systems must solve to build AGI.
- Sensory perception. Modern touch solutions offer AI tools more capabilities than ever, especially in home automation. Still, the system has a long way to go before AI has the same sensory abilities as humans.
- Fine motor skills. Many common activities require manual dexterity. We understand how difficult it is to program a robot arm to perform as accurately as a human arm. Not everyone is yet ready for robotic manipulators to cut our hair, perform medical procedures, or search us at the border.
- Socialization and emotional intelligence. Humans may subtly sense context, whereas our current AI cannot understand or consider emotions when generating responses.
Modern AI solutions cannot effectively establish connections between domains. At the same time, people may apply knowledge gained in one industry to other activities and adapt their theoretical knowledge to practical activities. At the same time, DL solutions must undergo training with vast amounts of data to process unfamiliar information efficiently.
Core AGI Applications
Real AGI systems are not yet in our life. However, there are several examples of narrow AI-backed solutions that partially approach human potential and sometimes even exceed it. Let’s look at some real examples.
- IBM Watson and other supercomputers utilize AI-backed instruments and significant computing power to solve complex scientific and engineering issues inaccessible to a standard computer. They analyze topics like dark matter or features of the human brain.
- Expert apps. Such AI-backed solutions replicate human thought processes, prescribe treatments based on diagnosis, and process molecular structures, creating additional decision-making opportunities.
- Large language model (LLM): gen AI pre-trained GPT models, including OpenAI solutions, such as ChatGPT-3 and ChatGPT-4, may replicate human language. Such solutions imitate human intelligence, composing sentences that are problematic to distinguish from human-generated text.
- Self-driving automobiles. AI-backed automobiles recognize cars, pedestrians, and objects and avoid them without violating traffic laws. They have impeccable perception, can make decisions instantly, and may act autonomously.
If you add artificial general intelligence to the above examples, their functionality may be significantly expanded. Thus, unmanned vehicles still require human control in critical situations. This is also true for creating songs, language algorithms, etc. These fields include the processes AI can manage and jobs requiring human interventions.
Final Words
The next decade will be critical for the development of AI solutions. Professionals are confident that the probability of building human-like AI systems by 2030 will be 25%. Increasing demand for robotic procedures and machine algorithms, combined with growing data volumes and advances in computing, form the basis for developing human-level AI solutions. It’s only a matter of time before advanced AI solutions from cutting-edge companies like Global Cloud Team become mainstream.
Top Articles
Leveraging Automation Integration to Build Successful Business
I am here to help you!
Explore the possibility to hire a dedicated R&D team that helps your company to scale product development.

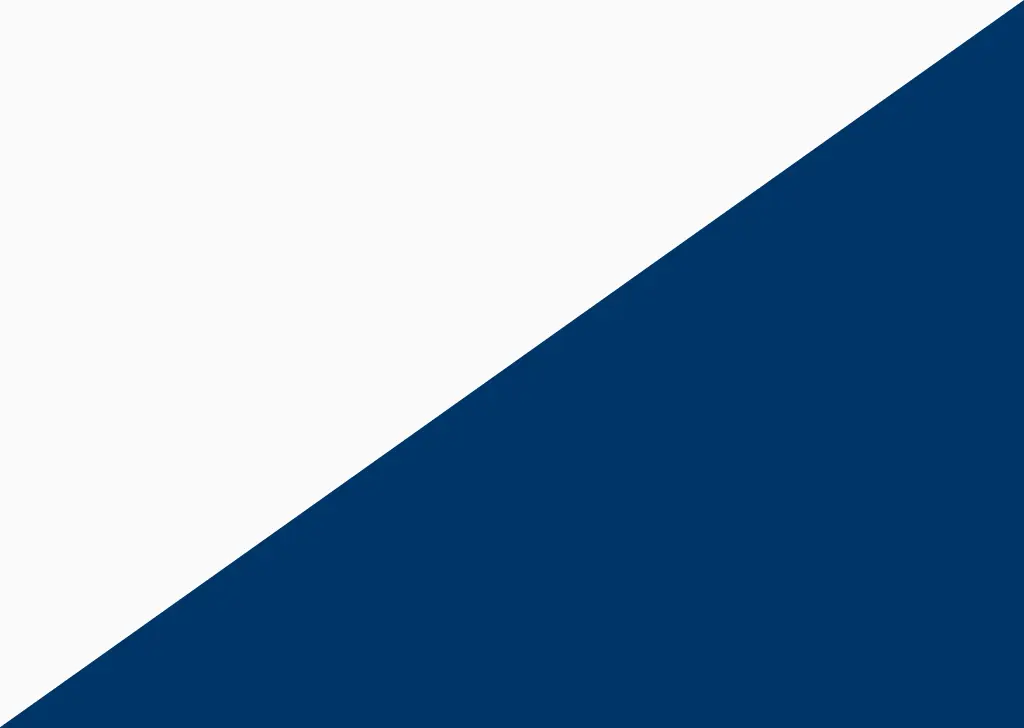